Research
INNVA1/2020/83
Assessment and transfer of deep-learning results of positron emission tomography neuroimaging analysis.
In this project a lot of software results of the group have been valorized so these results can be transferred to the companies interested in them. These results allow interested companies to make significant advances in the instrumentation dedicated to the analysis and diagnosis of neurodegenerative diseases, specifically in Alzheimer’s disease.
The group led by Dr. Luis Martí Bonmatí of the Instituto de Investigación Sanitaria La Fe (IIS La FE) is collaborating with us to optimize diagnostic efficiency.
This project is funded by Agencia Valenciana de la Innovació.
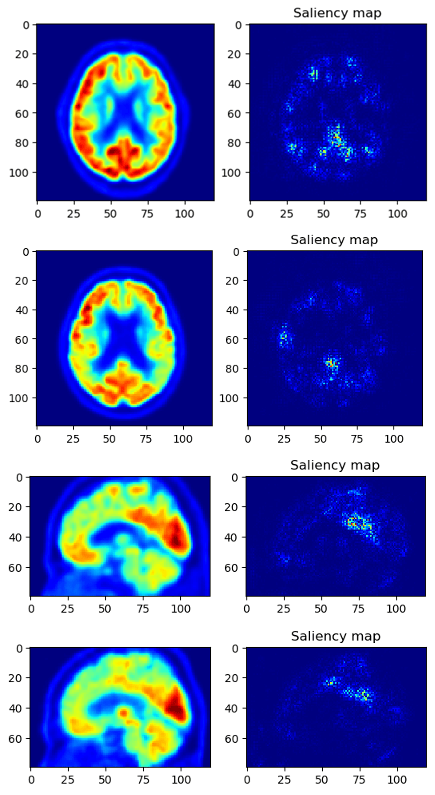
Mapas de activación de la red para imágenes no-Alzheimer (columna izquierda) y Alzheimer (columna derecha) obtenidos de la red
Objectives
The main objective of this project is the validation of the brain image processing software designed by our group. This software is able to analyze brain images by Deep-learning. The main features of this software are the following:
- It can be adapted to any PET scanner that is being developed or has already been developed, it is an added value for any product that the company already has developed.
- It allows the analysis of positron emission tomography images, including the automatic classification of images through Deep-learning,
- Initially it is developed for automatic classification of medical brain images for the prevention of neurological diseases.
- In the future it could be extended, with little additional work, to classification of other types of PET images such as cardiac images.
Results:
The software has been tested and modified according to the specifications of our collaborators at IIS La Fe.
The result has been the validation of a deep-learning (DL) FDG PET imaging algorithm capable of identifying patients with any neurodegenerative disease (Alzheimer’s Disease (AD), Frontotemporal Degeneration (FTD) or Dementia with Lewy Body (DLB)) among patients with Mild Cognitive Impairment (MCI).
Our model has an accuracy of 79 %, a sensitivity of 88 % and a specificity of 71 % in identifying patients with neurodegenerative diseases analyzed, achieving an area under the receiver operating characteristic curve (AUC) of 0.90. In external validation, the model retained a balanced accuracy of 80 %, sensitivity of 75 %, specificity of 84 % and an AUC of 0.86.
This binary classifier model based on FDG PET imaging enables early diagnosis of neurodegenerative diseases in patients with MCI in standard clinical settings with a balanced overall classification accuracy of 80%.
Potential market:
The potential market for the final product, once implemented in the relevant industry, are all hospitals and research centers that use positron emission tomography to study neurological imaging. Specifically, the final market (the recipients of the product once it is commercialized by the industry) are hospitals in charge of prevention, early diagnosis, monitoring of neurological diseases, research centers and pharmaceutical industries dedicated to the theranostics of neurological diseases.
It has been proven that the results are independent of the PET scanner with which the image was taken; it is fully adaptable to any PET scanner.
If you are interested please contact us
María José Rodríguez Alvarez mjrodri@i3m.upv.es
Funded by:
